자연어 처리(NLP)의 기초와 NLTK(Natural Language Tookit) 라이브러리 활용법에 대해서 알아보겠습니다. NLTK는 파이썬에서 가장 널리 사용되는 자연어 처리 라이브러리 중 하나로, 텍스트 분석과 처리를 위한 다양한 도구와 리소스를 제공 합니다.
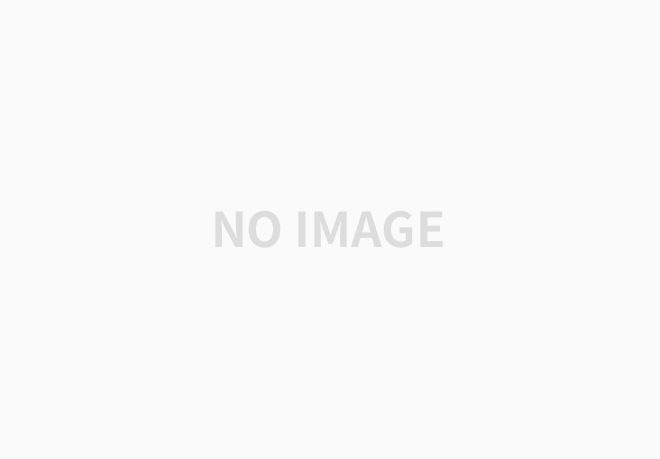
NLTK 소개
NLTK는 2001년 처음 개발된 이후 지속적으로 발전해왔으며, 텍스트 처리, 분류, 토큰화, 형태소 분석, 구문 북석 등 다양한 자연어 처리 작업을 지원 합니다. 이 라이브러리의 주요 특징은 다음과 같습니다.
풍부한 언어 데이터셋 제공
다양한 자연어 처리 알고리즘 구현
교육 및 연구 목적에 적합한 설계
활발한 커뮤니티 지원
상세한 문서화와 튜토리얼 제공
NLTK 설치하기
NLTK를 사용하기 위해서는 먼저 설치가 필요합니다. 파이썬이 이미 설치되어 있다면, 터미널이나 명령 프롬프트에서 다음 명령어로 NLTK를 설치할 수 있습니다.
pip install nltk
설치가 완료되면, 필요한 데이터를 다운로드 받아야 합니다. 파이썬 인터프리터에서 다음 코드를 실행하세요.
import nltk
nltk.download('punkt')
nltk.download('averaged_perceptron_tagger')
nltk.download('wordnet')
NLTK 주요 기능 살펴보기
1.토큰화(Tokenization)
토큰화는 텍스트를 더 작은 단위(토큰)로 나누는 과정입니다. NLTK는 단어와 문장 수준의 토큰화를 지원 합니다.
from nltk.tokenize import word_tokenize, sent_tokenize
text = "NLTK is a leading platform for building Python programs to work with human language data. It provides easy-to-use interfaces to over 50 corpora and lexical resources such as WordNet."
# 문장 토큰화
sentences = sent_tokenize(text)
print(sentences)
# 단어 토큰화
words = word_tokenize(text)
print(words)
2.품사 태킹(Part_of-Speech Tagging)
품사 태깅은 문장의 각 단어에 해당하는 품사를 할당하는 과정 입니다.
from nltk import pos_tag
tagged = pos_tag(words)
print(tagged)
3.명사 추출
텍스트에서 명사만 추출하고 싶을때 사용할 수 있습니다.
nouns = [word for word, pos in tagged if pos.startswith('N')]
print(nouns)
4.어간 추출(Stemming)
어간 추출은 단어의 어간 또는 어근을 추출하는 과정입니다. NLTK는 여러 종류의 스테머를 제공합니다.
from nltk.stem import PorterStemmer
stemmer = PorterStemmer()
stemmed_words = [stemmer.stem(word) for word in words]
print(stemmed_words)
5.표제어 추출(Lemmatization)
표제어 추출은 단어의 기본형을 찾는 과정 입니다. 어간 추출보다 더 정교한 결과를 제공합니다.
from nltk.stem import WordNetLemmatizer
lemmatizer = WordNetLemmatizer()
lemmatized_words = [lemmatizer.lemmatize(word) for word in words]
print(lemmatized_words)
6.불용어 제거(Stopword Removal)
불용어는 분석에 큰의미가 없는 일반적인 단어들 입니다. NLTK는 이러한 불용어 목록을 제공하여 텍스트에서 제거할 수 있게 해줍니다.
from nltk.corpus import stopwords
stop_words = set(stopwords.words('english'))
filtered_words = [word for word in words if word.lower() not in stop_words]
print(filtered_words)
NLTK를 활용한 간단한 텍스트 분석 예제
이제 위에서 배운 기능들을 조합하여 간단한 텍스트 분석을 해보겠습니다.
import nltk
from nltk.tokenize import word_tokenize
from nltk.tag import pos_tag
from nltk.stem import WordNetLemmatizer
from nltk.corpus import stopwords
def analyze_text(text):
# 토큰화
words = word_tokenize(text)
# 품사 태깅
tagged = pos_tag(words)
# 표제어 추출
lemmatizer = WordNetLemmatizer()
lemmatized = [lemmatizer.lemmatize(word) for word, tag in tagged]
# 불용어 제거
stop_words = set(stopwords.words('english'))
filtered = [word for word in lemmatized if word.lower() not in stop_words]
# 빈도 분석
freq_dist = nltk.FreqDist(filtered)
return freq_dist.most_common(10) # 상위 10개 단어 반환
sample_text = "Natural language processing (NLP) is a subfield of linguistics, computer science, and artificial intelligence concerned with the interactions between computers and human language, in particular how to program computers to process and analyze large amounts of natural language data."
result = analyze_text(sample_text)
print("Top 10 most frequent words:")
for word, frequency in result:
print(f"{word}: {frequency}")
이 예제는 주어진 텍스트를 토근화하고, 품사를 태깅하여, 표제어를 추출하고, 불용어를 제거한 후 가장 빈번하게 등장하는 10개의 단어를 출력 합니다.
마치며
NLTK는 자연어 처리르 위한 강력하고 유연한 도구입니다. 이 글에서는 NLTK의 기본적인 기능과 활용법에 대해 알아보겠습니다. NLTK를 사용하면 텍스트 데이터를 쉽게 처리하고 분석할 수 있으며, 이를 통해 다양한 NLP 작업을 수행할 수 있습니다.
NLTK의 더 많은 기능과 그급사용법을 익히고 싶다면, NLTK 공식문서와 튜토리얼을 참고하시기 바랍니다. 자연어처리는 계속해서 발전하는 분야이므로, 최신 트렌드와 기술을 따라가는 것도 중요 합니다.
NLTK를 활용한 자연어 처리 여정을 즐기시기 바랍니다.
'IT > Python' 카테고리의 다른 글
[Python] OpenCV를 이용한 이미지 처리 기초 (0) | 2025.01.21 |
---|---|
[Python] 파이썬으로 간단한 챗봇 만들기 (0) | 2025.01.21 |
[Python] TensorFlow를 이용한 딥러닝 모델 구현하기 (0) | 2025.01.20 |
[Python] Turtle 그래픽을 이용한 미니 게임 만들기 (0) | 2025.01.20 |
[Python] 파이썬을 이용한 퍼즐 게임 구현하기 (0) | 2025.01.19 |